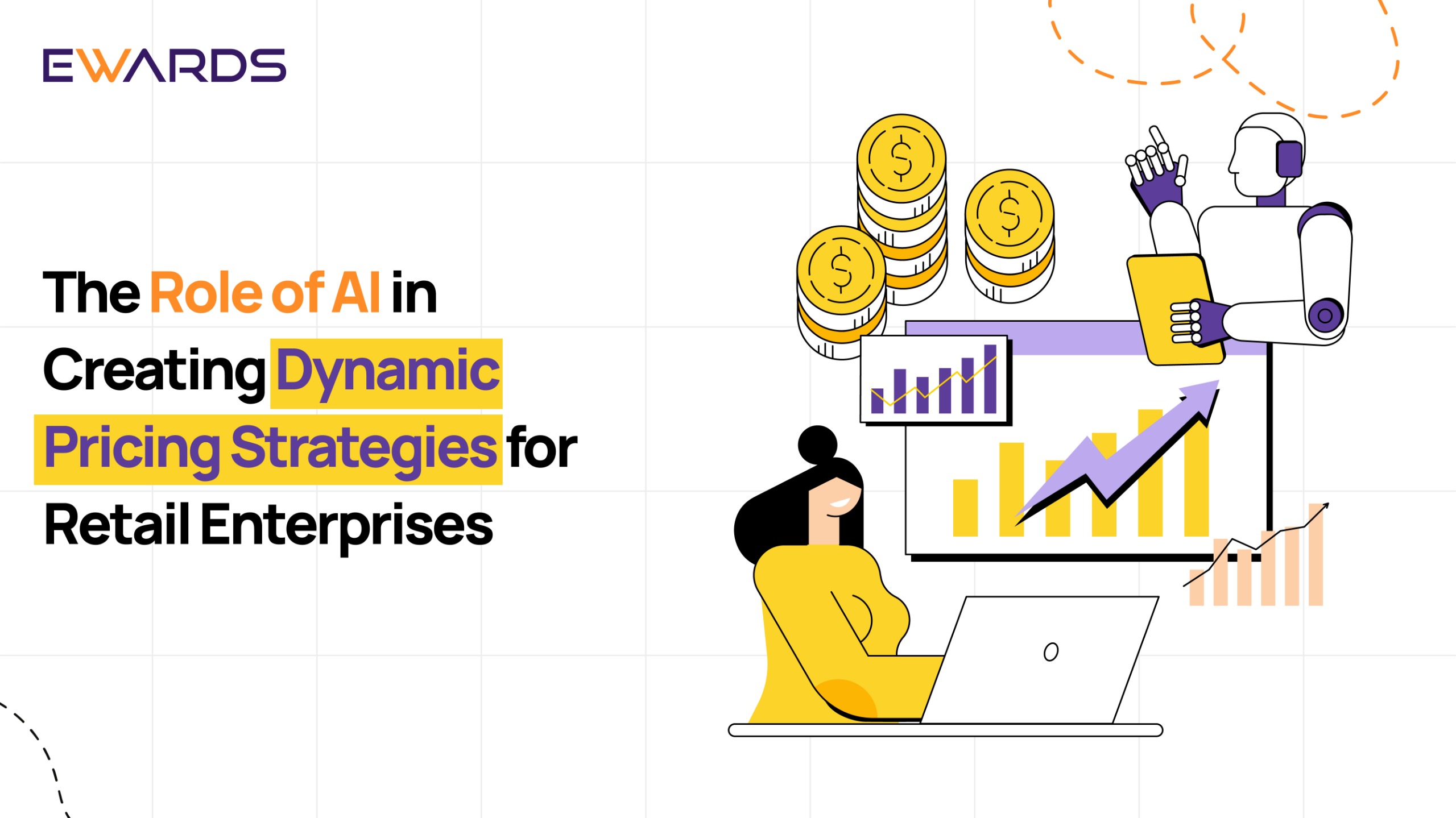
How confident are you that your pricing strategy is working for you, not against you?
Can your current pricing model keep up in a world where consumer expectations shift faster than trends? Or is it stuck in the past, reacting to market conditions instead of anticipating them? According to the current retail landscape, the traditional static pricing model is quickly becoming obsolete.
Imagine being able to automatically tweak your prices in real-time, adjusting them for seasonality, promotions, or stock levels—without lifting a finger. AI-powered dynamic pricing is the key. AI anticipates shifts, using real-time data to adjust prices continuously based on demand, competition, and even individual customer behavior.
The result? Pricing that’s not just competitive but maximized for both profit and customer loyalty.
Dynamic Pricing Strategy & How AI Powers It
Dynamic pricing is the strategy of continuously adjusting prices in response to real-time internal and external factors. Unlike static pricing (where the price of a product remains fixed for a longer time duration), dynamic pricing uses data-driven algorithms to determine the best price at any given time. The price adjustments are influenced by key factors like demand levels, competitor pricing, inventory stock, and broader market conditions.
In the AI-powered dynamic pricing strategy, Artificial Intelligence with Machine Learning analyzes vast sets of data along with learning patterns like seasonal demand shifts and price elasticity far faster and accurately than any human can. This means that pricing decisions that once took weeks of analysis can now be made in minutes across thousands of SKUs simultaneously.
Types of AI-Driven Dynamic Pricing Models
Different retailers have different goals, business objectives, and market dynamics, which is why different types of AI-driven dynamic pricing models are required. Some of them are:
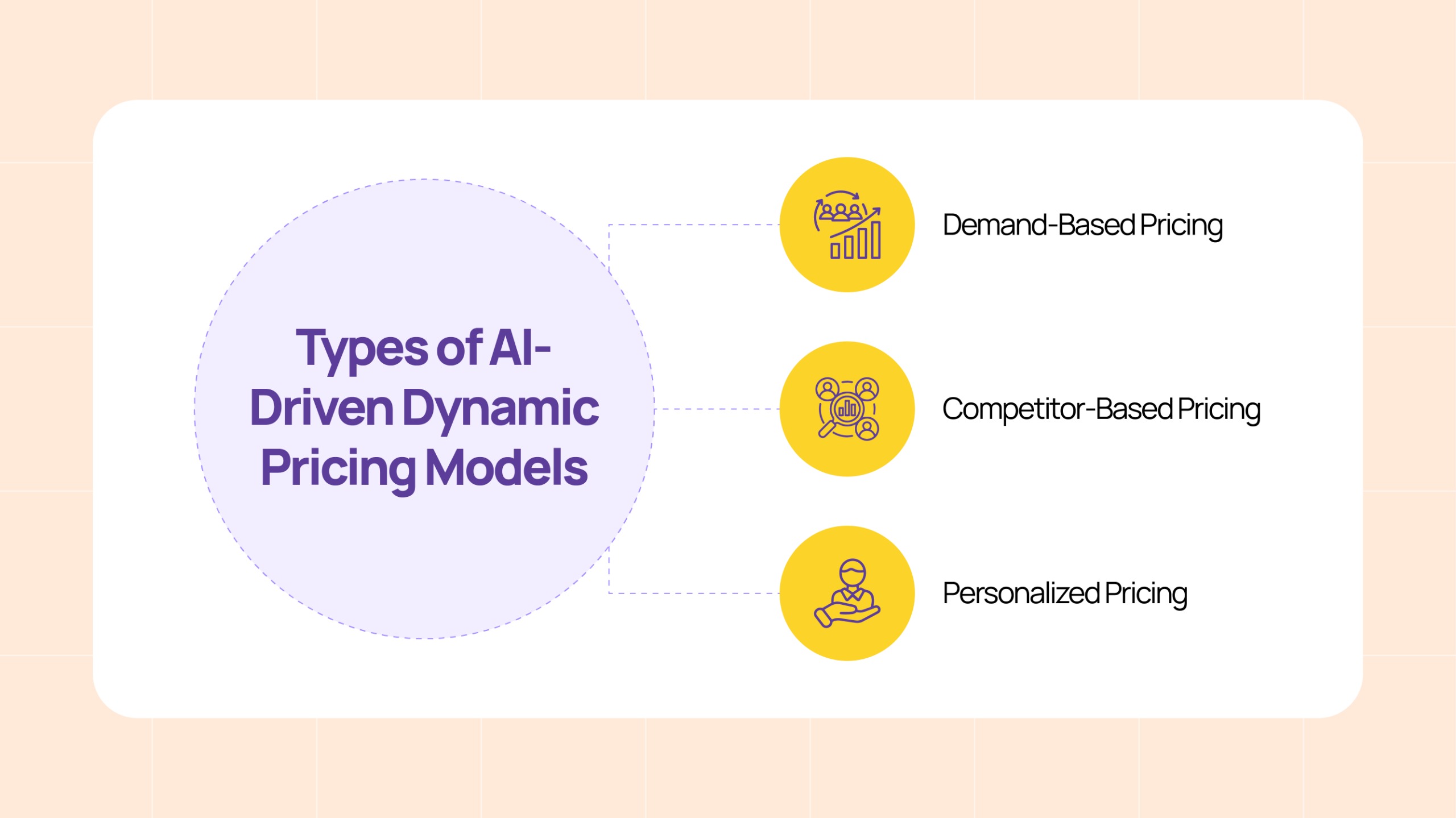
Demand-Based Pricing
Prices fluctuate according to the real-time demand of the customer. When demand spikes, prices can increase to capitalize on willingness to pay; when demand softens, prices drop to stimulate sales. This approach is akin to “surge pricing” and helps balance supply and demand – higher prices when items are hot, lower prices when they’re not. In retail, AI can imitate this model by instantly analyzing the sales velocity and stock levels to give a dynamic price.
Competitor-Based Pricing
According to this model, AI focuses on market competition, where it adjusts the price of your product in response to the pricing moves of your competitor. The basic idea of this model is to make sure that your offerings are attractively priced when compared to your competitors. AI-powered competitor monitoring tools scrape online prices and ensure retailers can react in near real-time.
This strategy is especially useful during big shopping events (think Black Friday or holiday sales) when customers aggressively compare prices – an AI can continuously match or beat key competitor prices to secure business.
Personalized Pricing
Retailers are also using AI to gauge the shopper’s willingness to pay and set the prices according to the individual customer or segment level. It makes use of data like browsing history, purchase frequency, loyalty status, and even demographics to tailor prices or discounts. AI algorithms segment customers and predict what pricing tactic will maximize conversion and lifetime value for each group with the idea of presenting the right price to the right customer – maybe offering a slight discount to price-sensitive shoppers while charging full price to those who have shown they’re willing to pay.
AI-driven dynamic pricing makes use of a customer-centric approach where prices are continuously optimized according to the market scenario rather than a cost-plus guess or a periodic manual update. The result is a pricing strategy that’s far more responsive and intelligent, which is increasingly vital in today’s fast-paced retail environment.
Benefits of AI-Based Dynamic Pricing in Retail
The adoption of an AI-powered dynamic pricing strategy can deliver significant advantages for retailers, impacting both the top line and bottom line:
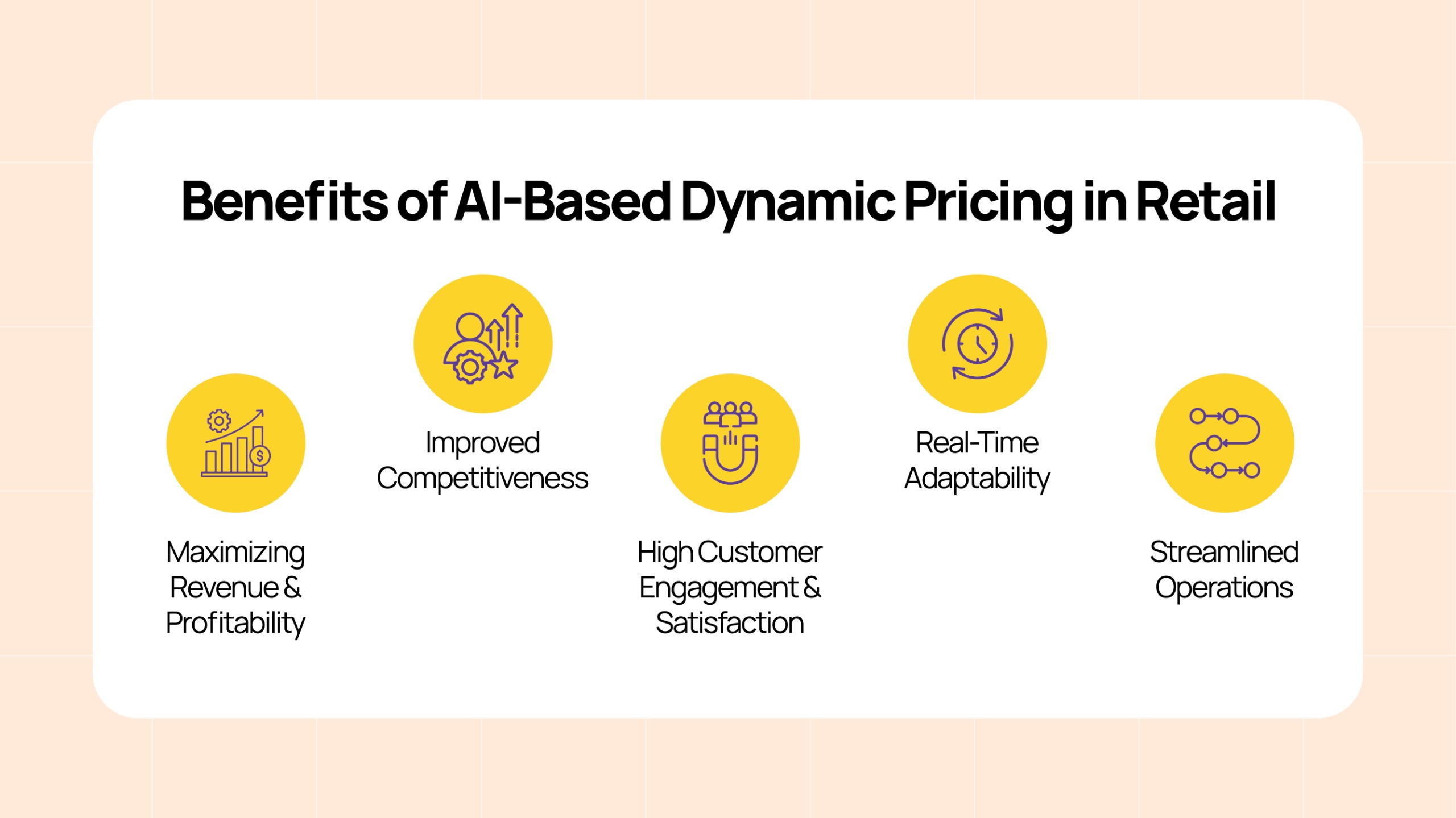
- Maximizing Revenue & Profitability – According to Accenture, implementing personalized and dynamic pricing strategies can boost revenue by up to 15% and expand profit margins by up to 5% (Hubspot). AI makes use of data to gain insights about customer purchasing behavior, competitor strategy, and market demands to come up with the most profitable price points. This closes the room for guesswork and creates an opportunity to capture untapped revenue.
- Improved Competitiveness – The retail landscape has changed drastically, where prices have become transparent and customers are quick to compare. The AI-powered dynamic pricing strategy gives retailers a competitive edge where they can maintain their prices in line with market conditions at all times. The algorithm present can instantly match or beat competitors’ pricing on key products, which would prevent revenue loss to rivals.
- High Customer Engagement & Satisfaction – Personalization and perceived fairness make dynamic pricing a boost for customer loyalty and satisfaction. AI-driven pricing can personalize promotions, which makes the customers feel valued and catered to. According to industry data, aligning prices closely with customer preferences leads to higher customer satisfaction scores; thus, by ensuring frequently viewed or high-traffic items remain competitively priced, retailers improve customers’ overall trust that they’re getting a good value.
- Real-Time Adaptability – Machine Learning is consistently learning from real-time data, and with the AI-pricing model, retailers can stay one step ahead of the marketing shifts. The result is a more resilient and agile pricing strategy that can handle volatility much better than static pricing. The BCG data supports this strategy because it has been observed that retailers who have already implemented AI-based dynamic pricing have achieved a gross profit increase of 5-10% with sustainable revenue growth.
- Streamlined Operations – Traditionally, pricing thousands of items across dozens of stores or an entire e-commerce catalog is an enormous manual task – teams of analysts crunch spreadsheets, and by the time prices are updated, the data might be outdated. AI automation removes much of this manual burden. Prices can be adjusted continuously without a human having to touch each SKU, freeing up the pricing team to focus on strategy rather than rote updates. Automation also reduces errors – cases of mispriced items or inconsistent pricing between channels go down.
Challenges of Implementing Dynamic Pricing in Retail
While the benefits are compelling, implementing AI-driven dynamic pricing is not without challenges. Retailers must navigate technical, organizational, and ethical hurdles to make the most of this strategy:
- Data Quality & Integration – The AI pricing model can only work with data from sales, inventory, competitors, consumer behavior, marketing trends, and more. These data are often stored in systems that cannot naturally talk to each other. This means that one of the major challenges in the implementation of dynamic pricing is creating unified, real-time data that can be fed to AI. Also, inconsistent and erroneous data can lead to faulty pricing decisions.
- Customer Trust and Perception – Customers are accustomed to stable pricing in retail; thus, when prices change dynamically, it is plausible that they will be treated unfairly. For instance, during the COVID-19 pandemic, Amazon’s algorithms raised prices on high-demand essentials like hand sanitizer and face masks, leading to accusations of price gouging. Therefore, transparent communication and an ethical pricing strategy are important for customer loyalty.
- Regulatory Concerns – Several industries are subject to strict pricing laws and consumer protection standards. Businesses should implement pricing algorithms according to such standards as failure to meet compliance can result in penalties, legal disputes, and even reputational damage.
- Implementation Complexity & Black Box Algorithms – There is also the challenge of integrating the AI solution with existing pricing processes and systems (ERP, POS, e-commerce backend). According to McKinsey, even after the tech is in place, one common hurdle is the “black box” nature of AI algorithms. The mathematics of machine learning models can be very opaque, where it can churn out pricing recommendations without a simple explanation of why that price is optimal.
Implementation Strategies
To be able to successfully integrate the dynamic pricing strategy, it is important to make use of a well-planned approach, which is listed below:
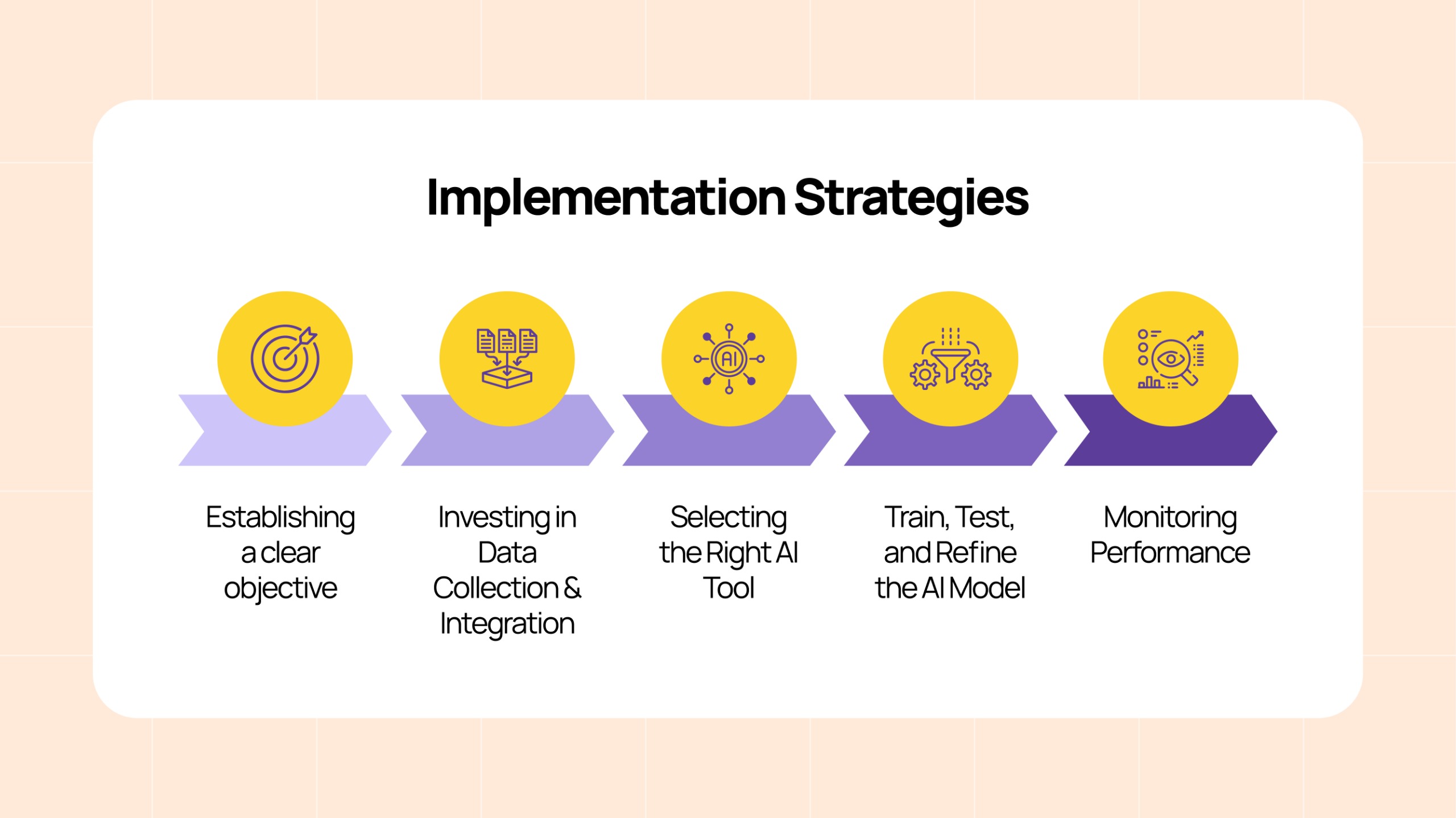
- Establishing a clear objective – It is important to set goals and objectives to get the anticipated results from the implementation. Having clear objectives and rules upfront will guide the AI model’s design and prevent extreme outcomes.
- Investing in Data Collection & Integration – Retailers can also conduct a data audit to determine what internal and external data they can leverage. Internal data would include historical sales data, web analytics, inventory levels, and any relevant customer data. External data includes competitor pricing information and other macro factors like holidays, weather, or local events if they affect your sales. Integrate these data sources into a single platform to be accessible by the AI system.
- Selecting the Right AI Tool – Next, it is important to decide the technology approach depending on your company’s resources and expertise. Off-the-shelf price optimization solutions offer ready-made AI that can be configured according to your needs, which might be quicker to deploy. Alternatively, if you have a strong data science team, you might build a tailored model that gives you more flexibility. In either case, evaluate options through the lens of your objectives.
- Train, Test, and Refine the AI Model – Before making the dynamic pricing strategy live, it is important to thoroughly train and test the system. For this, retailers need to feed the AI model with historical data to learn patterns of demand, seasonality, and price elasticity. The model should be trained to predict outcomes like sales volume at different price points. Once trained, conducting a back-test is a necessity where AI generates pricing recommendations using past data and sees how those would have performed versus your actual outcomes.
- Monitoring Performance – Once dynamic pricing is in production, set up dashboards to track metrics like revenue, margin, price change frequency, and inventory levels in real time. If the AI suggests any extreme price changes, have a review process. Over time, as trust in the system grows, you can automate more. Additionally, update the AI with fresh data and retrain it as needed because consumer behavior and competitor strategies evolve, and your model should, too.
AI in Dynamic Pricing Success Stories
Amazon
This e-commerce giant is the key user of the dynamic pricing strategy. It sets its pricing algorithms to adjust item prices incessantly — up to millions of times per day — to respond to demand and competitor prices. From McKinsey’s data, Amazon uses AI to ensure that it offers the lowest price on popular, high-visibility items while recouping margin on niche products that are less price-sensitive. Its AI monitors competitors (on and off Amazon’s marketplace), inventory levels, and even user browsing behavior to optimize prices in real time.
Uber
Uber, a ride-hailing app, is also very well-known for its dynamic pricing strategy. Depending upon the day, time, distance, traffic in your route, and current rider-to-driver demand, Uber uses dynamic pricing algorithms. Thus, the cost of a trip on a Tuesday night would be different from the cost of the same trip on Saturday night.
Conclusion
Dynamic pricing powered by AI offers significant advantages for retail companies looking to increase their bottom line, enhance customer loyalty, and stay ahead of competitors. Retailers who are not yet leveraging AI in pricing strategies are missing out on the chance to not only increase profits but also provide a tailored and seamless shopping experience for their customers.
Are you ready to make your pricing as smart as your customers?